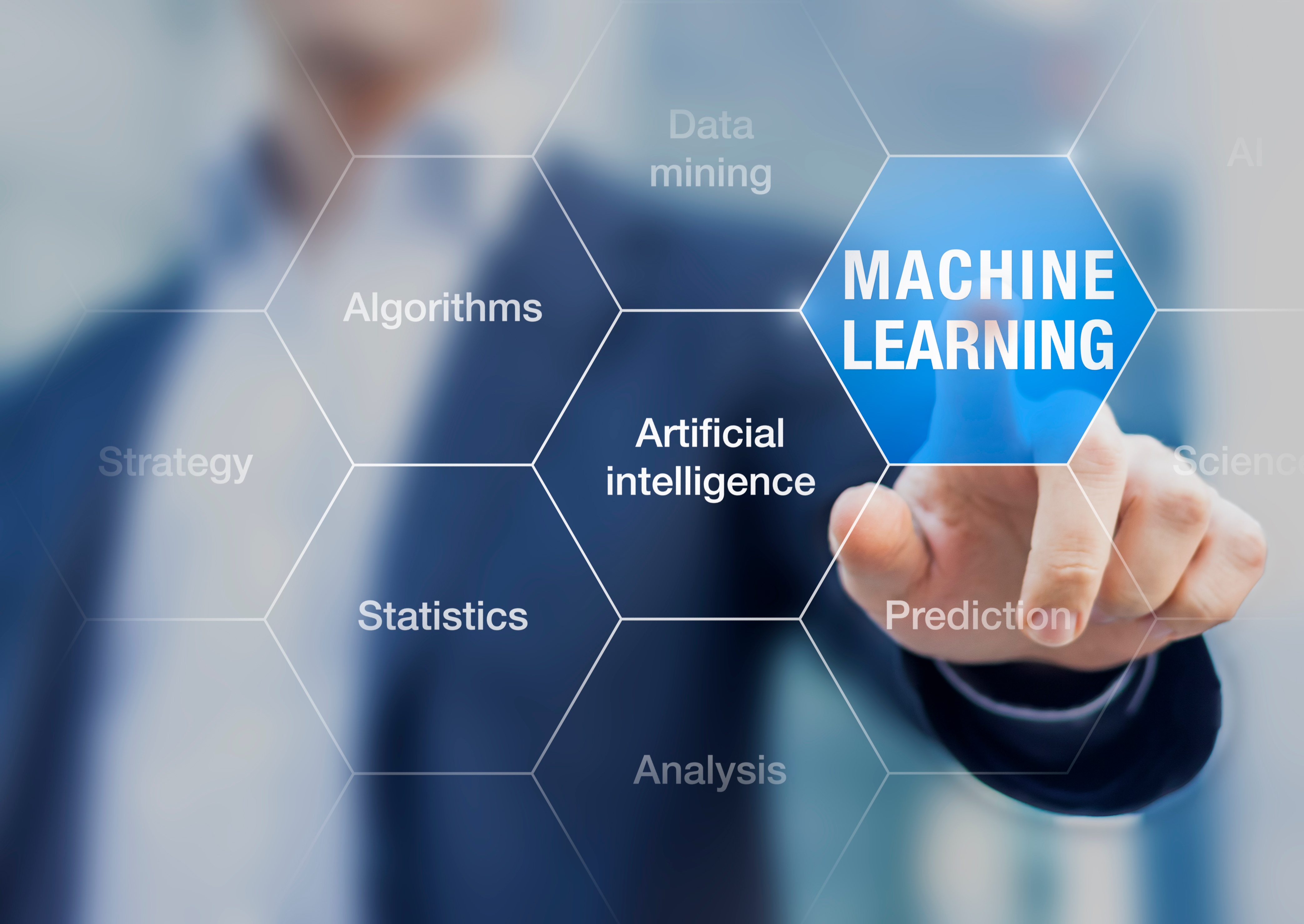
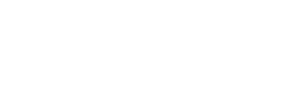
What Does Machine Learning Mean for the Healthcare Revenue Cycle?
There’s no denying that Big Data is everywhere in our day-to-day lives. By some estimates, there has been more data created in the past 2 years than in all of human existence combined.
The rapid evolution of technology is aiding the vast amount of choices we have in the consumer world to leverage machine learning in an effort to study our habits and predictively deliver what we are looking for, when and where we need it.
Ever wonder how those shoes that you’ve been shopping for on the web suddenly shows up in your Facebook newsfeed? Guess what, that’s Machine Learning.
So how does Machine Learning leverage those same algorithms to impact healthcare? Well the answer is that it varies depending on whether we are talking about direct patient care or driving back office efficiencies.
With direct patient care, there are encouraging applications involving learning and problem solving that will lead to faster and more accurate diagnose for improved outcomes. However, we will always rely on the judgement of the care giver to make the final call.
When looking at the back office of healthcare and specifically the financial operations of the organization, the human factor is greatly reduced. We are sorting through tons of transactional data to arrive at a single defensible truth.
On the flip side, the human factor can contribute to errors and missed opportunities for collecting what’s owed to the organization. With the right application, Machine Learning can address many of these back office challenges.
The important questions that must be addressed are:
AM I GETTING REIMBURSED FOR THE PROPER AMOUNT ACCORDING TO MY CONTRACT?
DID I ACCOUNT FOR THE POSSIBILITY OF OTHER VARIANCES?
To arrive at the answer to these questions, there are many analytics that Machine Learning can simplify. Here are two that could be considered low hanging fruit:
- Use an algorithm to spot trends for contractual variances such as add-on reimbursable items not paid, CPT codes not paid on claims, identify patients that should have been covered under a different plan, etc.
- Identify false variances that can lead to better registration practices.
These improvements in productivity can reduce payment delays and denials.
The goal is to leverage machine learning analytics to catch these mistakes and get them corrected automatically reversing claims that have been denied inappropriately.
For example, let’s say that a patient goes to the ER with stomach pains. The hospital registrar asks for their insurance information and sees they have the HMO plan with a co-pay of $100. Then, the registrar gets distracted by another patient and accidently chooses the PPO plan in the registration system instead. The PPO rate was $95, so now the hospital will be underpaid by $5.
Doesn’t sound like much, right? Let’s put this in perspective, though.
According to Healthcare Business Insights, research shows that roughly 30-40% of denials are caused by registration errors, representing as much as 0.5% in lost revenue.
For a hospital with $500M in revenue, that’s worth $2.5M. That’s $2.5M that could have been spent to fund projects like patient engagement, hospital facility renovations, salary increases, and so much more.
How does your system catch these variances today? Is it a manual review? Why not leverage an algorithm to look for these variances and spotlight them to improve efficiencies, reduce cycle time, and impact the financial health of your organization?
Soon, Machine Learning will be as synonymous in healthcare as it is in our everyday lives. Big Data is driving the challenge and it’s never been more cumbersome than in the back office.
How can your organization make the most out of your Big Data?
About Fred Sheffield
Mr. Sheffield is a top-producing senior sales leader with over 20 years of challenger sales development, talent management and verifiable results in surpassing sales targets and client expectations in the information systems and healthcare technology industries. Mr. Sheffield is accustomed to developing teams to craft business-building visions with senior level executive management and steering negotiations with c-level executives and key decision makers within top healthcare organizations and Fortune 500 B2B companies. He is a motivational consensus builder, skillful at calling teams to action and solving sales challenges through training and mentorship to elevate performance, customer satisfaction, profitability and shareholder value. Mr. Sheffield previously led successful sales teams at SAVO Group and CareFusion.